Phylogenetics methods
Erick Matsen
Thank you to: Trevor Bedford (Fred Hutch)
“data”: sequence alignment
A sequence alignment is not a fact
… it is a complex inference.
In molecular phylogenetics, homology means…
In molecular phylogenetics, homology means…
Thus sequence alignment for phylogenetics is
a super
challenging inference. More about this later.
Types of phylogenetic inference methods
- Distance-based
- Parsimony
- Likelihood-based
- Maximum likelihood
- Bayesian
Distance-based phylogenetics
Distance-based phylogenetics
Note that the matrix doesn’t have to come from sequence data.
Parsimony phylogenetics
Parsimony
is based on Occam’s
razor
Among competing hypotheses that predict equally well, the one with
the fewest assumptions should be selected.
The next few slides are from Trevor Bedford.
Say we have three viruses
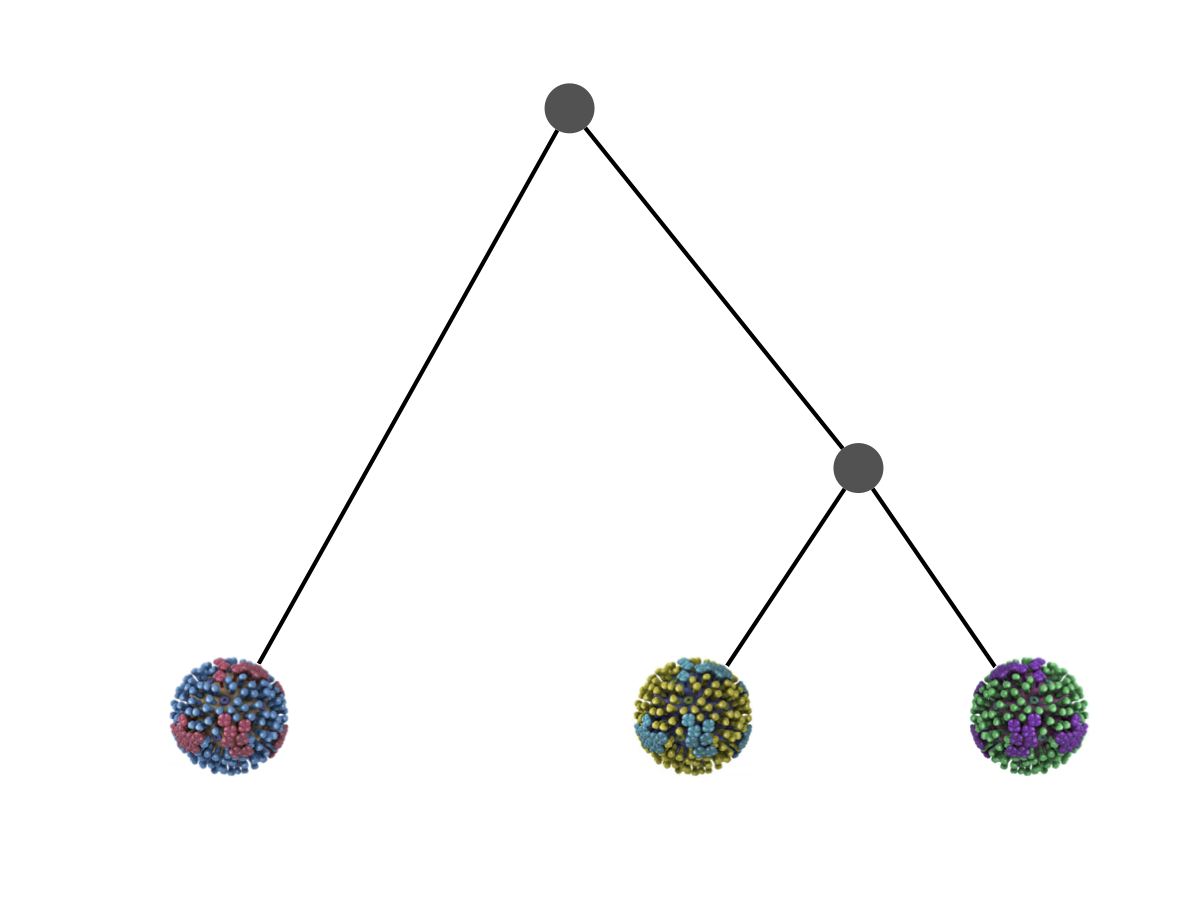
Say we have three viruses
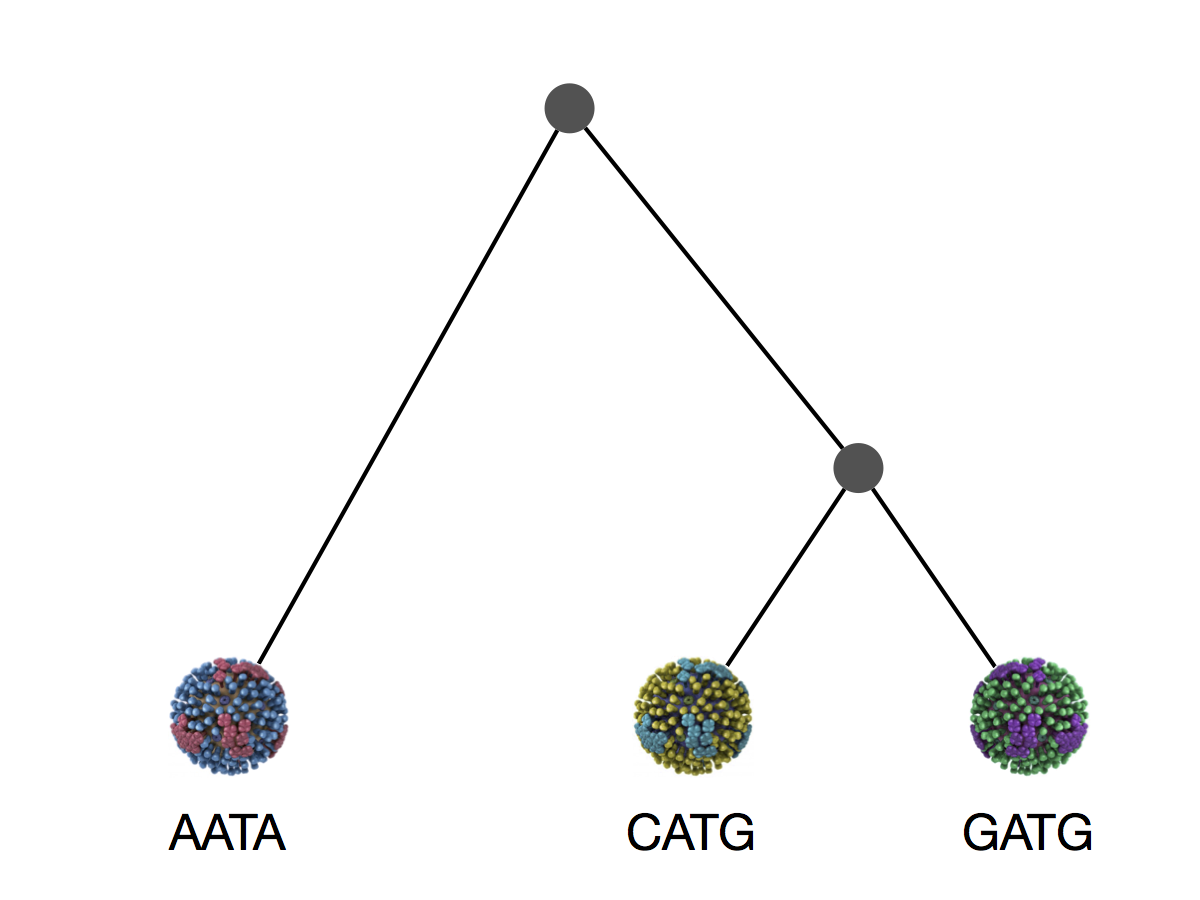
We can explain these sequences with 3 mutations
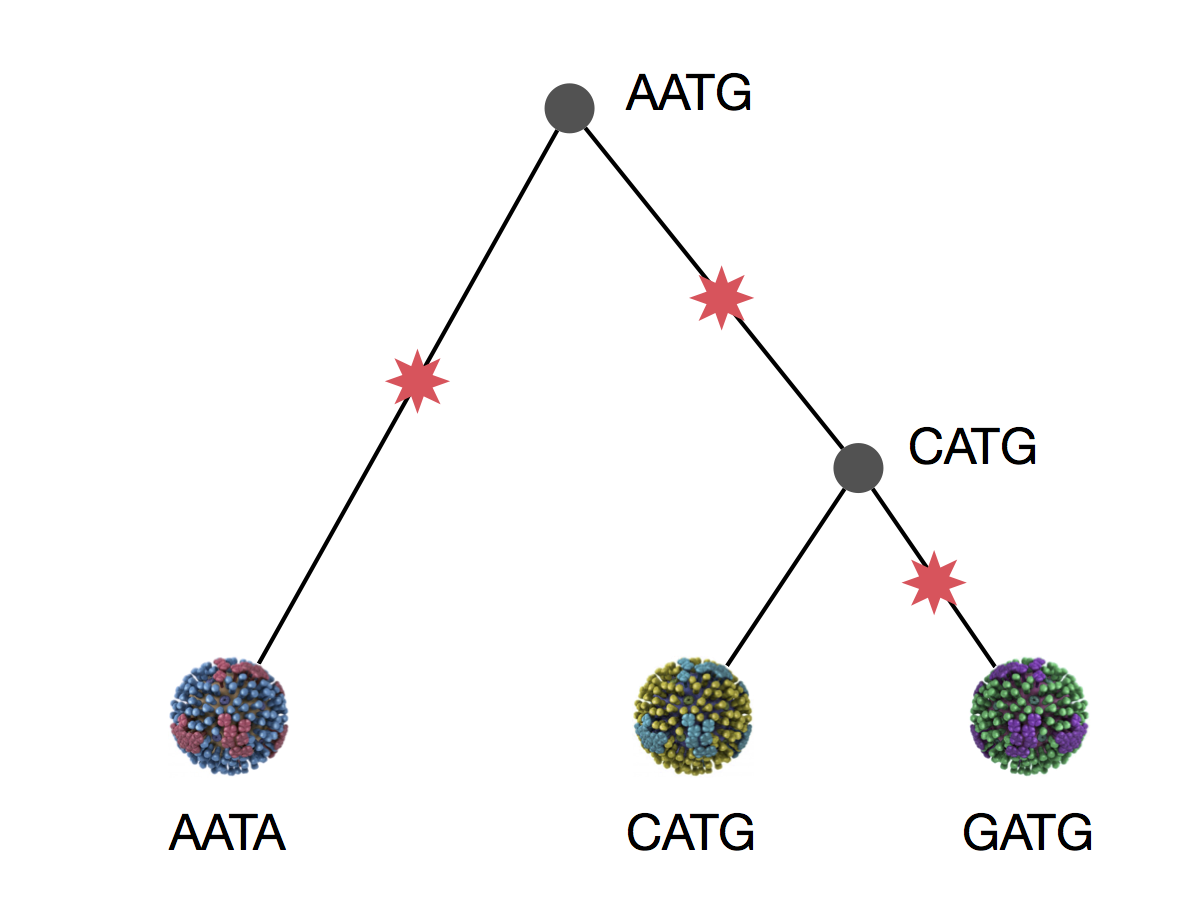
This topology requires 3 mutations at minimum
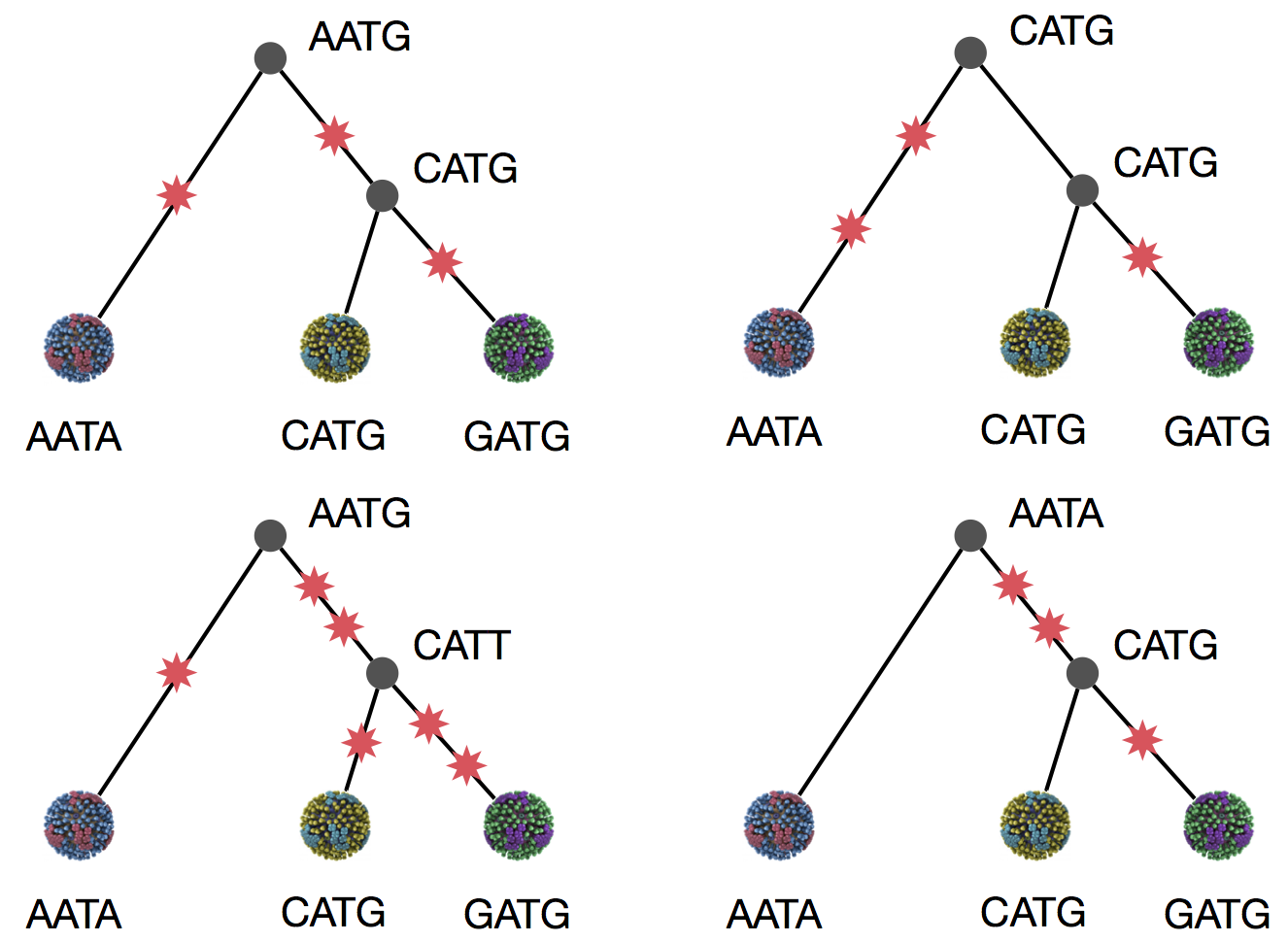
Exercise: which topology is more parsimonious?
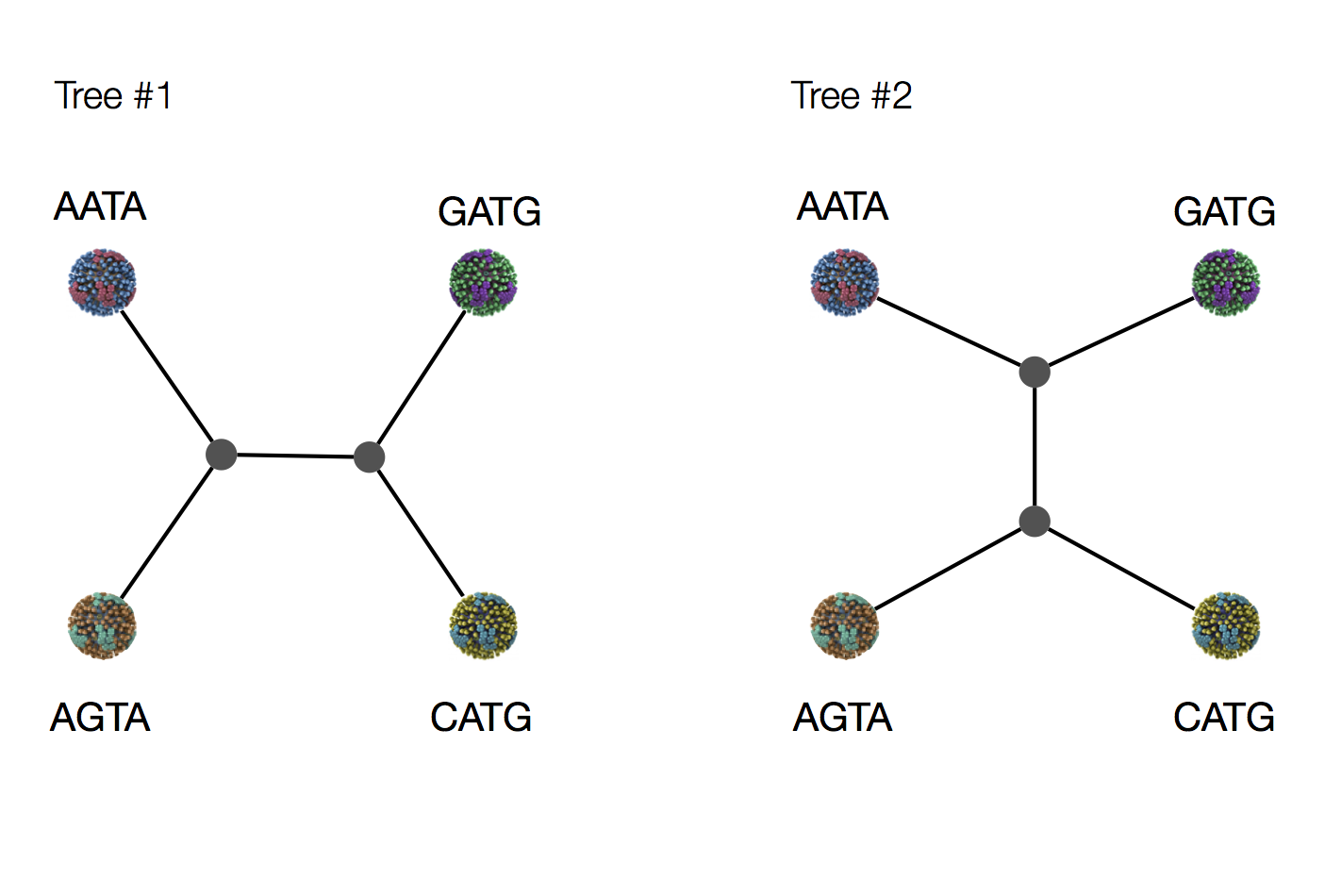
Exercise: which topology is more parsimonious?
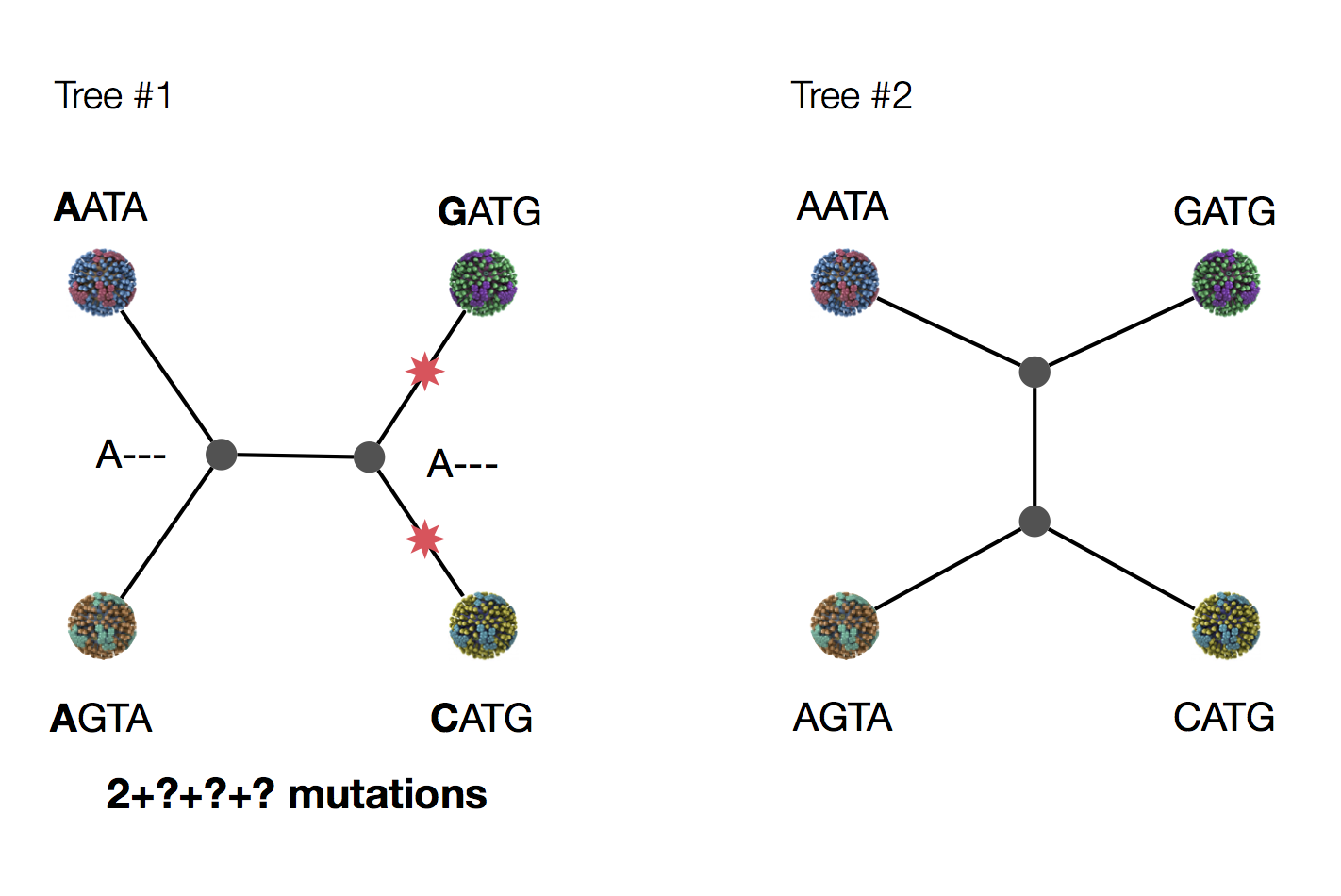
Exercise: which topology is more parsimonious?
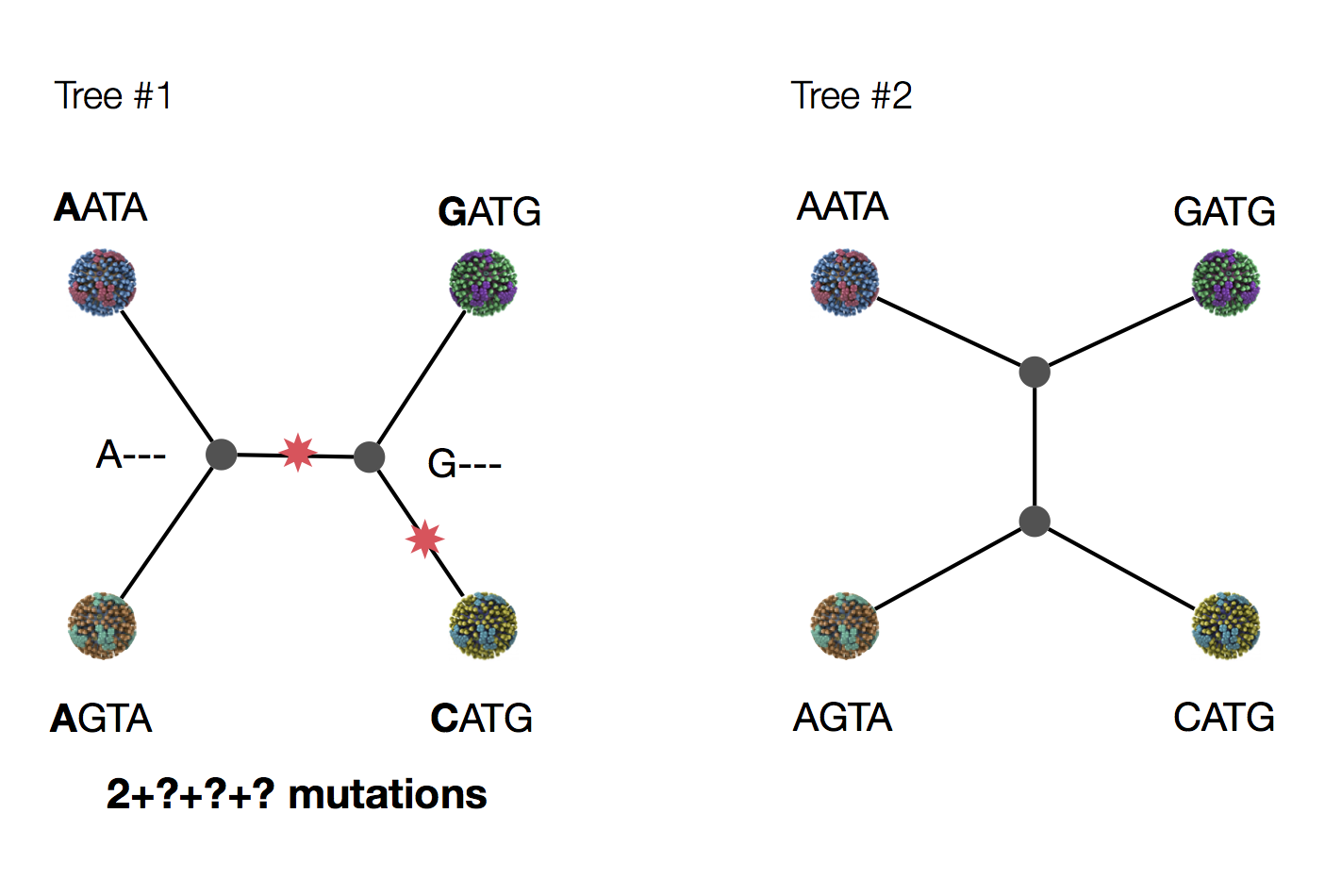
Exercise: which topology is more parsimonious?
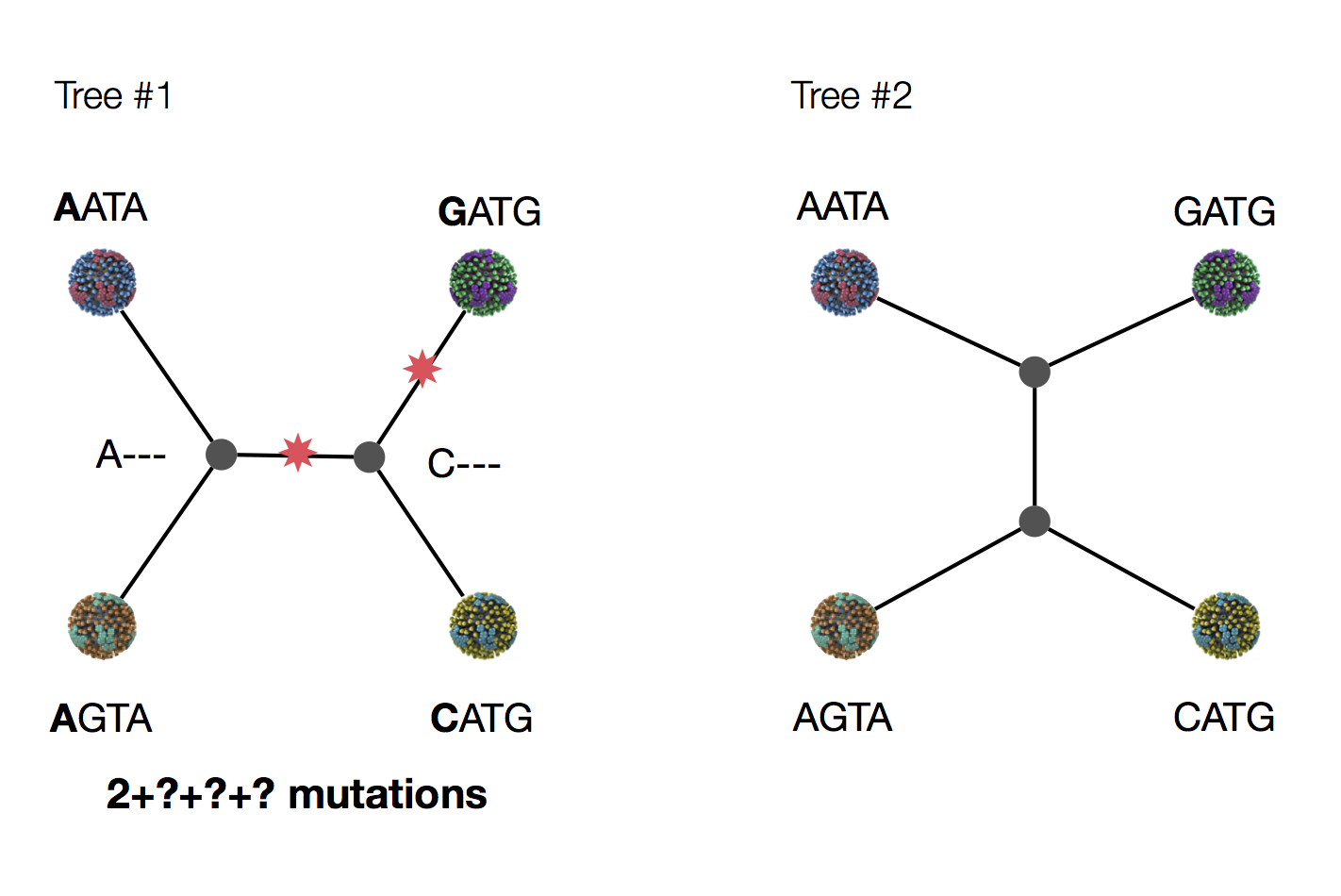
Exercise: which topology is more parsimonious?
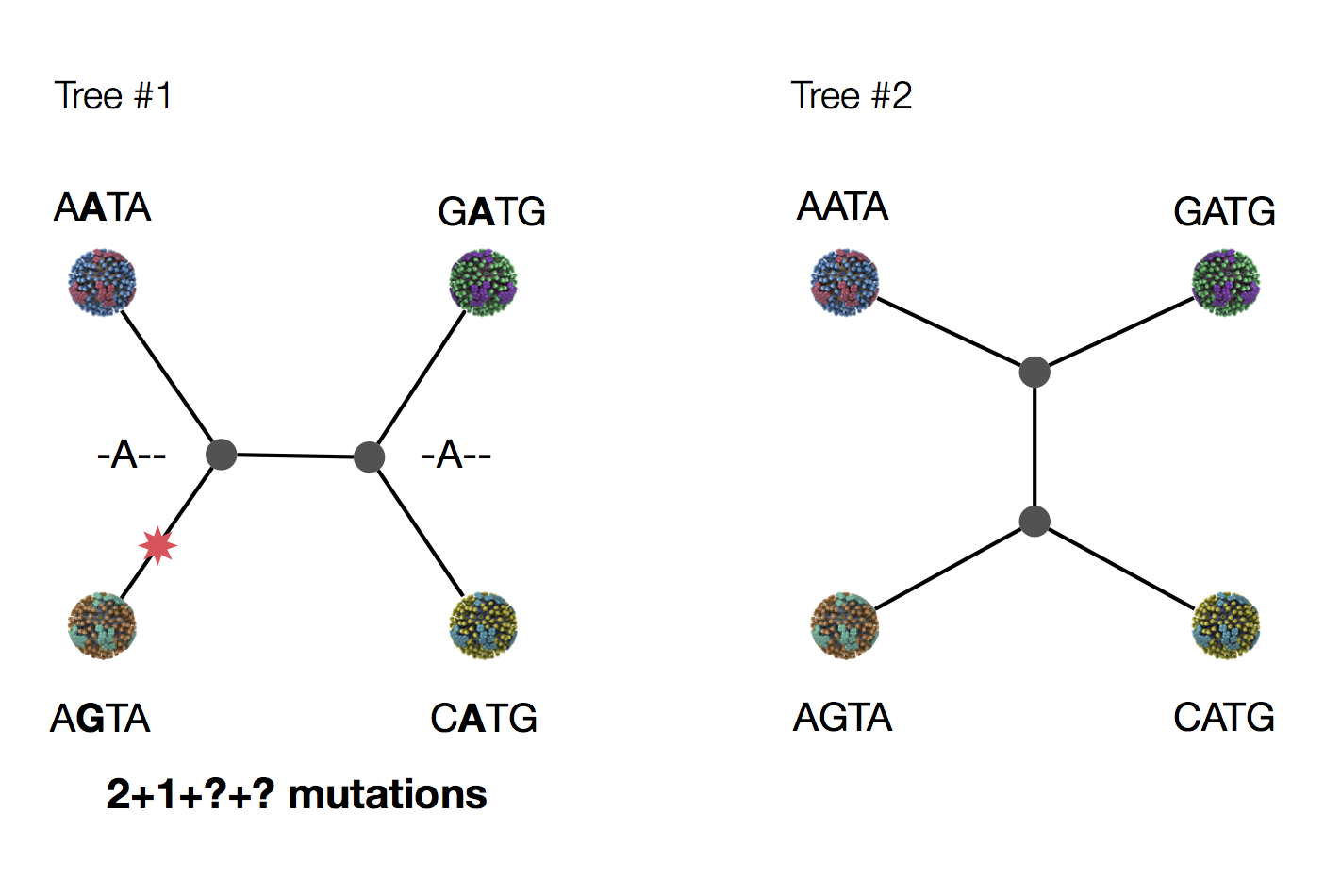
Exercise: which topology is more parsimonious?
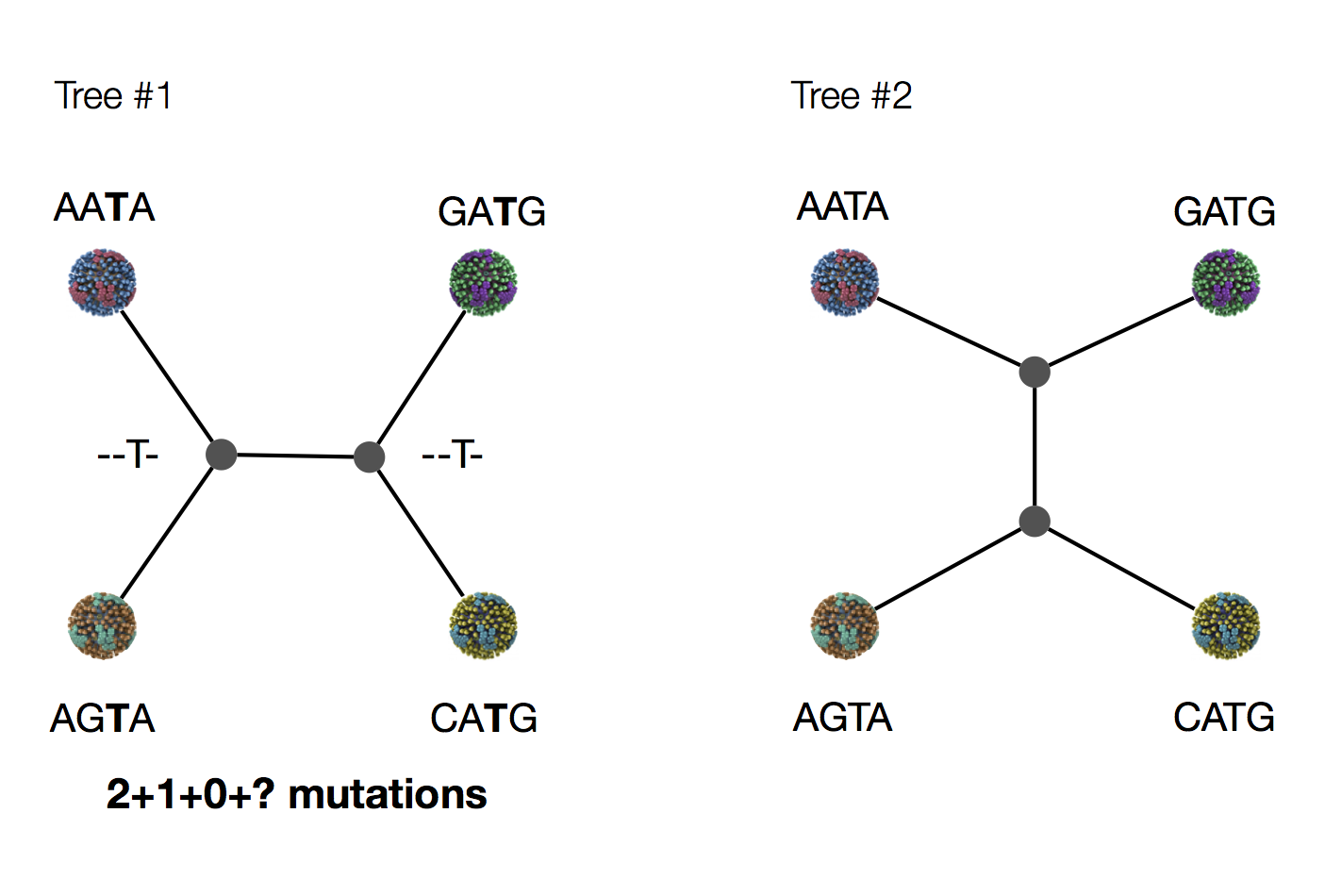
Exercise: which topology is more parsimonious?
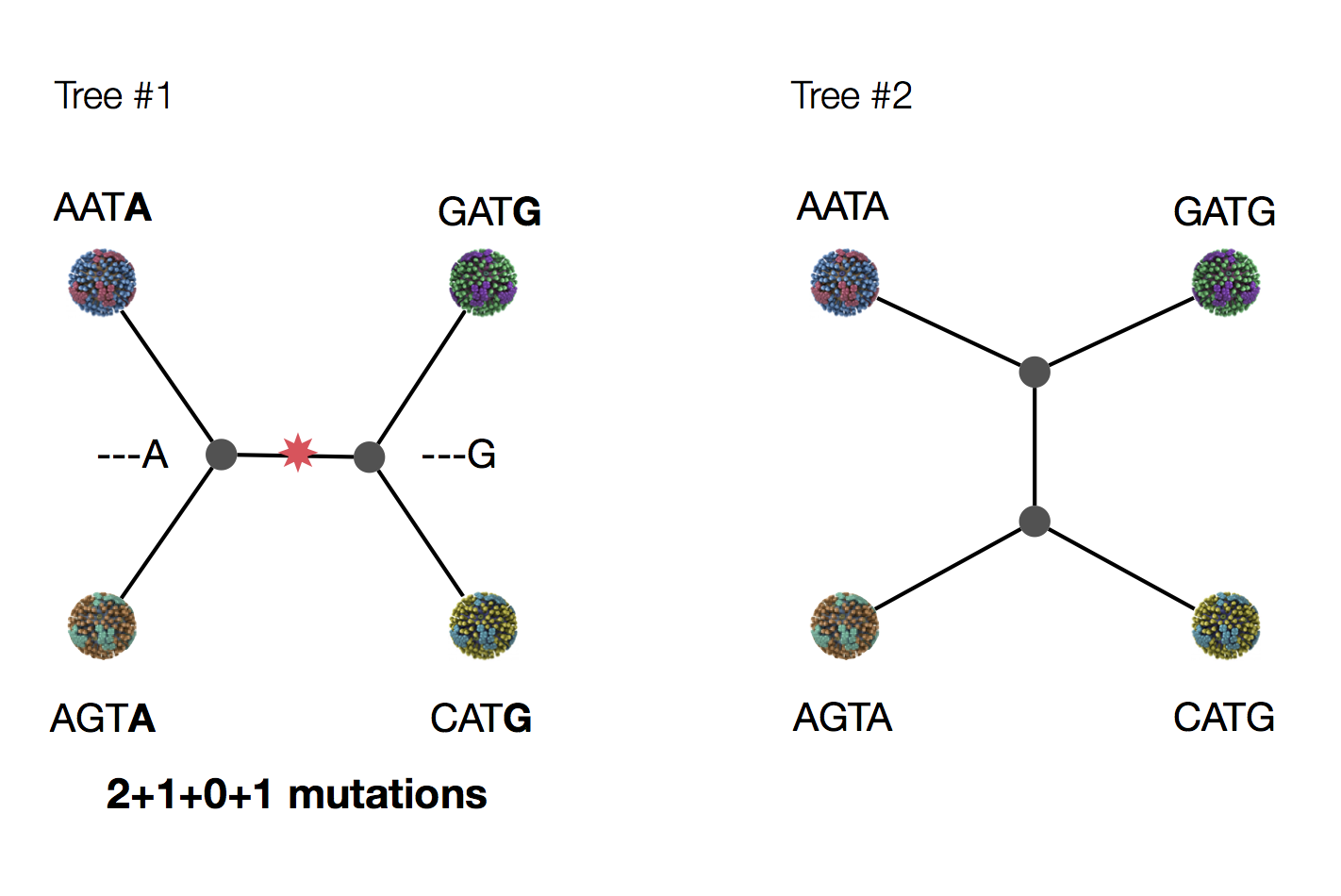
Exercise: which topology is more parsimonious?
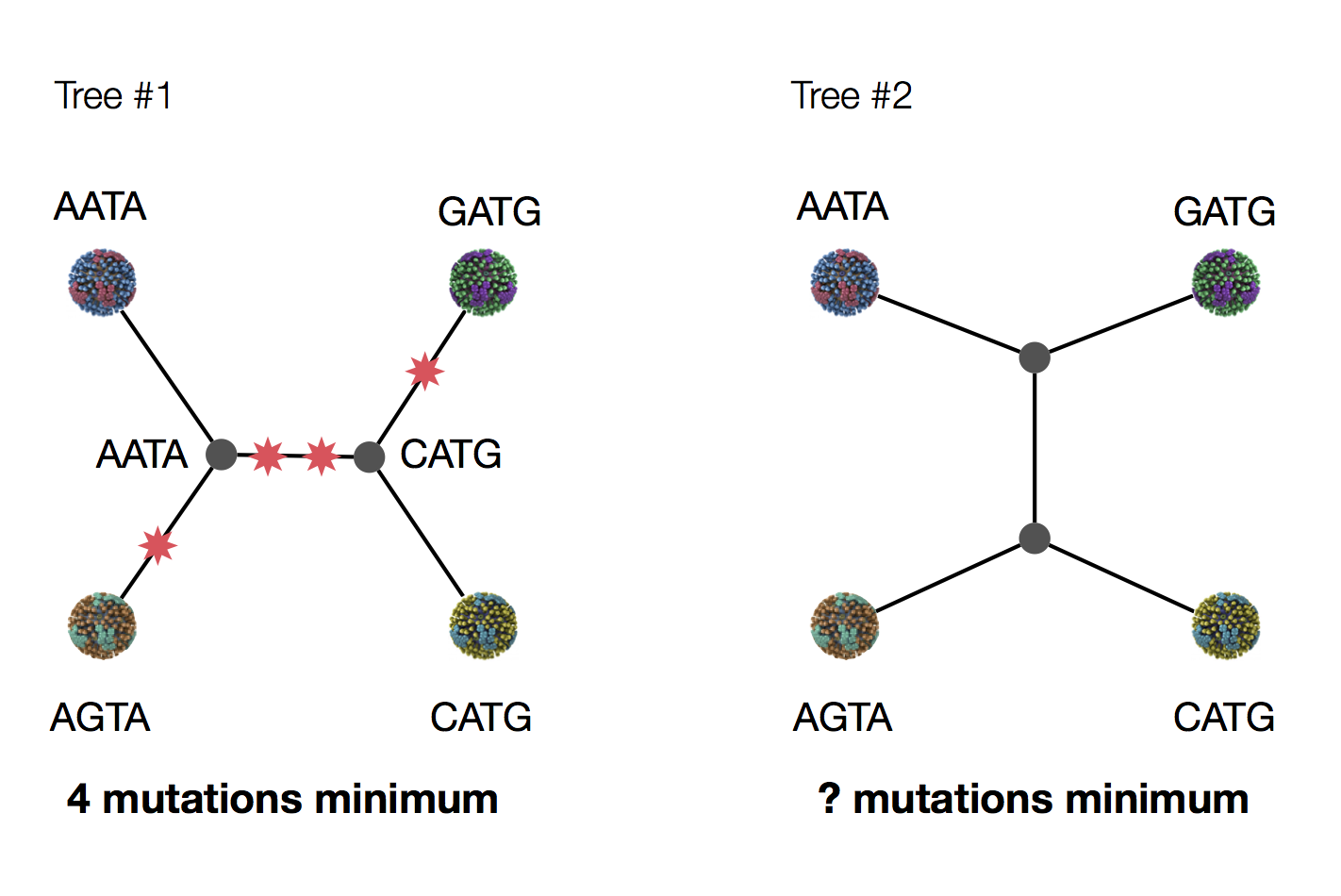
Parsimony seems sensible.
Is it the most popular phylogenetics method?
No. There are situations in which the correct tree has more
mutations.
Long branch attraction
http://slideplayer.com/slide/9059488/
Long branch attraction
http://slideplayer.com/slide/9059488/
Are we cooked?
Given enough data, likelihood methods will converge to the true tree.
Likelihood setup
-
Come up with a statistical model of experiment in terms of some data and
some parameters
-
Write down a likelihood function that expresses the probability
of generating the observed data given those parameters
-
Now we can evaluate likelihood under various parameter values
-
We will write this likelihood \(\Pr(D \mid
\theta)\).
Why is this appropriate notation?
Example: picking peaches
Say \(p\) is the probability of
getting a ripe peach, and each draw is independent.
-
What is the probability of getting two ripe peaches in a row?
-
What about if after harvesting two peaches, we have one ripe one?
-
What about if after harvesting 20 peaches, we have 6 ripe ones?
Example: picking peaches
Say that, after harvesting 20 peaches, we have 6 ripe ones.
Say \(p\) is the probability of
getting a ripe peach, and each draw is independent. Model using the binomial
distribution.
The likelihood of getting the observed result is \[
{ {20} \choose 6} \, p^6 \, (1-p)^{20-6}.
\] Recall: \({ {20} \choose 6}\)
is the number of ways of choosing 6 items out of 20.
Peach picking likelihood surface
The maximum likelihood estimate of the parameter(s) of
interest is the parameter value(s) that maximize the likelihood.
Questions
-
What is the maximum likelihood (ML) estimate of \(p\) given our experiment?
-
Would the result of this ML estimate be different if we got 60 ripe
peaches out of 200?
-
Intuitively, would the shape of the likelihood curve be different with
this larger dataset?
Likelihood recap
- Maximum likelihood is a way of inferring unknown parameters
- To apply likelihood, we need a model of the system under
investigation
- In general, the “likelihood” is the likelihood of generating the
data under the given parameters, written \(P(D
| \theta),\) where \(D\) is the
data and \(\theta\) are the
parameters.
Crazy but typical model assumptions
- differences between sequences only appear by point mutation
- evolution happens on each column independently
- sequences are evolving according to reversible models (this
excludes selection and directional evolution of base composition)
- the evolutionary process is identical on all branches of the
tree